Top 3 Uses of Machine Learning and AI in Finance
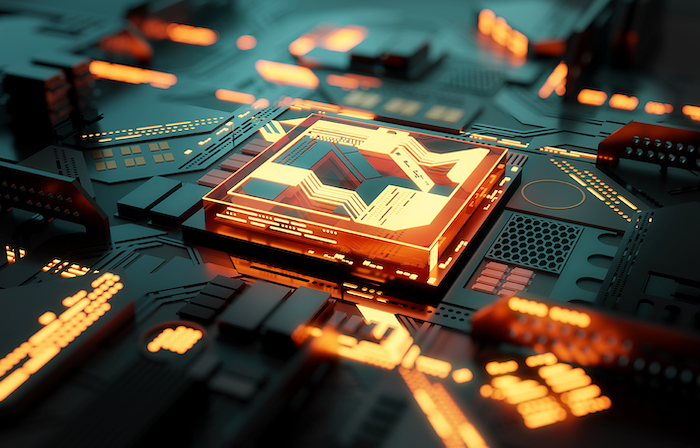
The term artificial intelligence might bring to mind ideas of science fiction rather than finance, but in reality, this technology is an integral part of the business world. Every single day, there are applications that rely on artificial intelligence technology to calculate trades and finalize transactions, and the impact of such programs reaches beyond mere business. These three examples of how AI operates in finance provide that it is more than just a trend, and it probably plays a bigger role in your life than you realize.
Financial Advisory
The field of financial advisory is shifting rapidly as millennials face an entirely different monetary circumstance than prior generations. Still, many young people are seeking wealth management advice, but not necessarily from a human consultant. Instead, they may connect with a so-called Robo-Adviser that can offer customized financial advice based on data provided by the customer. This application is powered by an AI that guides clients through the consultation.
The AI application then calculates its advice using historical data and algorithmic risk assessment. The result is custom-tailored financial advice without the cost of an in-person advisor. This is attractive to younger clientele who may not have substantial funds to consult about to begin with, but the AI still provides an opportunity to gain insight into a financial future and begin planning effectively.
Fraud Detection
Perhaps the most prolific work of Ai programs is in the field of fraud detection. Anywhere there is money, there is fraud, but smartly engineered AIs aim to put a stop to that. Some of the applications that most commonly utilize this technology include the following:
- Catching fake checks and documents
- Flagging suspicious transfers
- Recognizing patterns in transactions
AI in fraud detection works by utilizing machine learning to continuously identifying new characteristics of fraud and incorporate these into its algorithm for future application. Some of the techniques that an AI program may use to accomplish this include data mining, neural nets, expert systems, and link analysis in order to assess potential fraud on any transaction.
Sentiment Analysis
The concept of sentiment analysis is not necessarily concerned exclusively with finance—as the name suggests, this AI operation involves a semiotic analysis of the intent, tone, and implications of a text—whether it be a text, an email, or a tweet. An AI can analyze such content and produce a reading which determines whether the text in question is positive, negative, or neutral.
Though this can be applied to many fields, it is particularly relevant to finance, because a single tweet can crash a stock. It is important for investors and other financial professionals to understand what they’re up against when it comes to the discourse surrounding the products and services they’re trading. A sentiment analysis AI can help them accomplish exactly that.
Sentiment analysis further allows prediction of trends. An AI performing this operation can comprehensively analyze a massive body of content spanning years and produce a detailed analysis identifying time-sensitive trends of positive, negative, and neutral discourse. This insight can then be used to make stock projects or to simply better understand the subject in question.